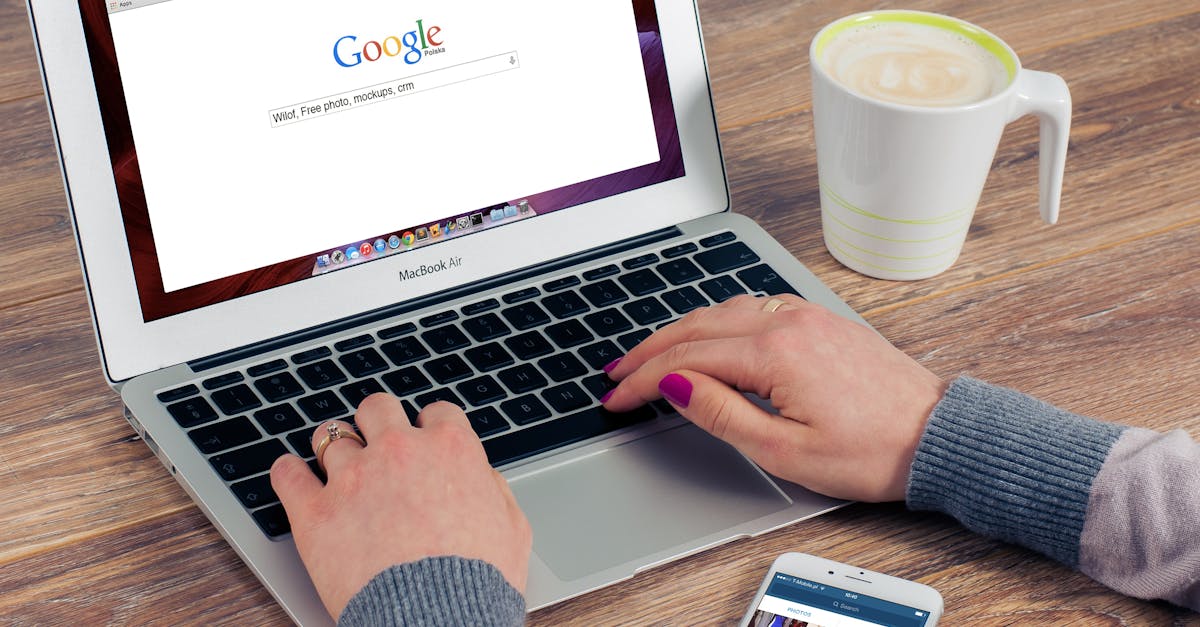
Table Of Contents
Techniques for Data Analysis
Techniques for data analysis play a pivotal role in the landscape of big data, influencing the way organisations interpret and utilise their information. Different approaches such as statistical analysis, machine learning, and data mining provide various insights into patterns and trends within large datasets. The choice of technique often depends on the specific goals of the organisation and the nature of the data being examined. While some techniques focus on descriptive statistics that summarise past events, others delve into predictive modelling to anticipate future outcomes.
The distinction between analytics and reporting is essential for organisations striving to enhance decision-making processes. Reporting typically provides straightforward summaries of data, highlighting key metrics through dashboards and visualisations. This approach helps stakeholders understand what has happened over a defined period. Conversely, analytics adds depth by examining the underlying factors, offering complex interpretations and insights that can illuminate the reasons behind trends or changes in the data. The combination of both techniques ensures that organisations not only track performance but also gain strategic insights that can drive future actions.
Analytical Approaches in Big Data
In the realm of big data, analytical approaches play a critical role in deriving meaningful insights from vast datasets. Techniques such as predictive analytics utilise historical data to forecast future trends, allowing businesses to make informed decisions. Descriptive analytics, on the other hand, focuses on understanding past performance through data aggregation and visualisation. Both methodologies are essential for effective Analytics and Reporting, serving different purposes in the decision-making process.
Moreover, prescriptive analytics takes it a step further by not only predicting outcomes but also recommending actions based on data analysis. Machine learning algorithms are often employed to enhance these analytical approaches, enabling systems to learn from data patterns and improve over time. These advanced techniques provide organisations with a comprehensive understanding of their data landscape, crucial for effective Analytics and Reporting strategies.
Speed of Data Processing
Data processing speed plays a crucial role in how organisations derive insights from big data. In many cases, the demands of business require timely access to information. This necessity can dictate whether data is processed in real-time or through batch processes. Real-time processing enables immediate analysis of incoming data, making it suitable for environments where rapid decision-making is essential. On the other hand, batch processing involves gathering data across set intervals, which can lead to delays but often allows for comprehensive analysis over larger datasets.
The choice between real-time and batch processing ultimately affects the effectiveness of both analytics and reporting. Real-time systems present the advantage of up-to-the-minute insights, fostering agility and responsiveness. Conversely, batch processing can provide more detailed reports at set intervals, useful for routine analysis and decision-making. Understanding the implications of processing speed is integral for organisations seeking to optimise their data strategies and enhance overall performance in their big data initiatives.
Realtime vs. Batch Processing
Real-time processing involves the continuous input and analysis of data as it becomes available. This method allows organisations to react swiftly to changes and emerging trends, making it essential for applications requiring immediate insights. In contrast, batch processing collects data over a period before analysis occurs. This approach is often more efficient for processing large volumes of data that do not require instant feedback.
In the realm of Analytics and Reporting, the choice between real-time and batch processing hinges on the specific needs of an organisation. Real-time analytics provides up-to-the-minute insights, beneficial for dynamic environments such as finance or e-commerce. Batch processing, meanwhile, can be more suitable for in-depth reporting, allowing for comprehensive analysis over larger datasets with less urgency. Each method plays a crucial role in shaping how organisations leverage big data to drive decision-making.
Tools and Software
The landscape of tools and software for data analysis has evolved to meet the diverse needs of organisations looking to harness big data. Popular platforms such as Tableau and Power BI provide robust functionalities for visualising data and generating comprehensive reports. These tools allow users to convert vast quantities of raw data into understandable insights, facilitating better decision-making. Integration with other systems ensures that data flows seamlessly, enhancing the overall efficiency of Analytics and Reporting processes.
In addition to visualisation tools, numerous software options cater specifically to data mining and predictive analytics. Platforms like Apache Spark and Hadoop are essential for users seeking to process large datasets efficiently. They deliver powerful capabilities for running complex analytical queries, enabling stakeholders to derive actionable insights from their data. With the right software, the distinction between Analytics and Reporting becomes more manageable, as organisations can track performance in real-time while also exploring long-term trends.
Popular Platforms for Reporting and Analytics
Numerous platforms cater to the diverse needs of Analytics and Reporting. Tools like Tableau and Power BI enable users to visualise data and create interactive dashboards, making the analysis accessible. These platforms often come with user-friendly interfaces, allowing even those with limited technical skills to derive insights from complex datasets. Their capabilities extend beyond simple data displays, providing functionalities like real-time data connectivity and the ability to drill down into specifics.
On the other hand, platforms such as Google Data Studio and Klipfolio focus on reporting, offering features that allow businesses to present data in a clear and concise manner. These tools are designed to help organisations monitor key performance indicators (KPIs) effectively. With customisable templates and automation features, users can generate reports that are both informative and visually appealing, streamlining the process of sharing insights across teams.
FAQS
What is the main difference between reporting and analytics in big data?
The main difference lies in their purpose; reporting is primarily about presenting data in a structured format for easy consumption, while analytics involves deeper examination and interpretation of data to uncover patterns and insights.
How do reporting and analytics complement each other in big data?
Reporting provides the foundational data that can be used for analysis, while analytics allows businesses to derive actionable insights from that data, making both processes essential for informed decision-making.
What are some common tools used for reporting in big data?
Common tools for reporting include Tableau, Microsoft Power BI, and Google Data Studio, which allow users to create visualisations and dashboards that summarise data effectively.
Can analytics be performed in real-time?
Yes, analytics can be performed in real-time or in batch processing modes, depending on the requirements of the business and the tools being used.
Why is speed of data processing important in analytics?
Speed is crucial in analytics because timely insights can significantly impact decision-making processes, especially in fast-paced industries where conditions can change rapidly.